Last year, a major focus of my research was developing a better understanding of threat models for AI risk. This post is looking back at some posts on threat models I (co)wrote in 2022 (based on my reviews of these posts for the LessWrong 2022 review).
I ran a survey on DeepMind alignment team opinions on the list of arguments for AGI ruin. I expect the overall agreement distribution probably still holds for the current GDM alignment team (or may have shifted somewhat in the direction of disagreement), though I haven’t rerun the survey so I don’t really know. Looking back at the “possible implications for our work” section, we are working on basically all of these things.
Thoughts on some of the cruxes in the post based on developments in 2023:
- Is global cooperation sufficiently difficult that AGI would need to deploy new powerful technology to make it work? – There has been a lot of progress on AGI governance and broad endorsement of the risks this year, so I feel somewhat more optimistic about global cooperation than a year ago.
- Will we know how capable our models are? – The field has made some progress on designing concrete capability evaluations – how well they measure the properties we are interested in remains to be seen.
- Will systems acquire the capability to be useful for alignment / cooperation before or after the capability to perform advanced deception? – At least so far, deception and manipulation capabilities seem to be lagging a bit behind usefulness for alignment (e.g. model-written evals / critiques, weak-to-strong generalization), but this could change in the future.
- Is consequentialism a powerful attractor? How hard will it be to avoid arbitrarily consequentialist systems? – Current SOTA LLMs seem surprisingly non-consequentialist for their level of capability. I still expect LLMs to be one of the safest paths to AGI in terms of avoiding arbitrarily consequentialist systems.
In Clarifying AI X-risk, we presented a categorization of threat models and our consensus threat model, which posits some combination of specification gaming and goal misgeneralization leading to misaligned power-seeking, or “SG+GMG→MAPS”. I still endorse this categorization of threat models and the consensus threat model. I often refer people to this post and use the “SG + GMG → MAPS” framing in my alignment overview talks. I remain uncertain about the likelihood of the deceptive alignment part of the threat model (in particular the requisite level of goal-directedness) arising in the LLM paradigm, relative to other mechanisms for AI risk.
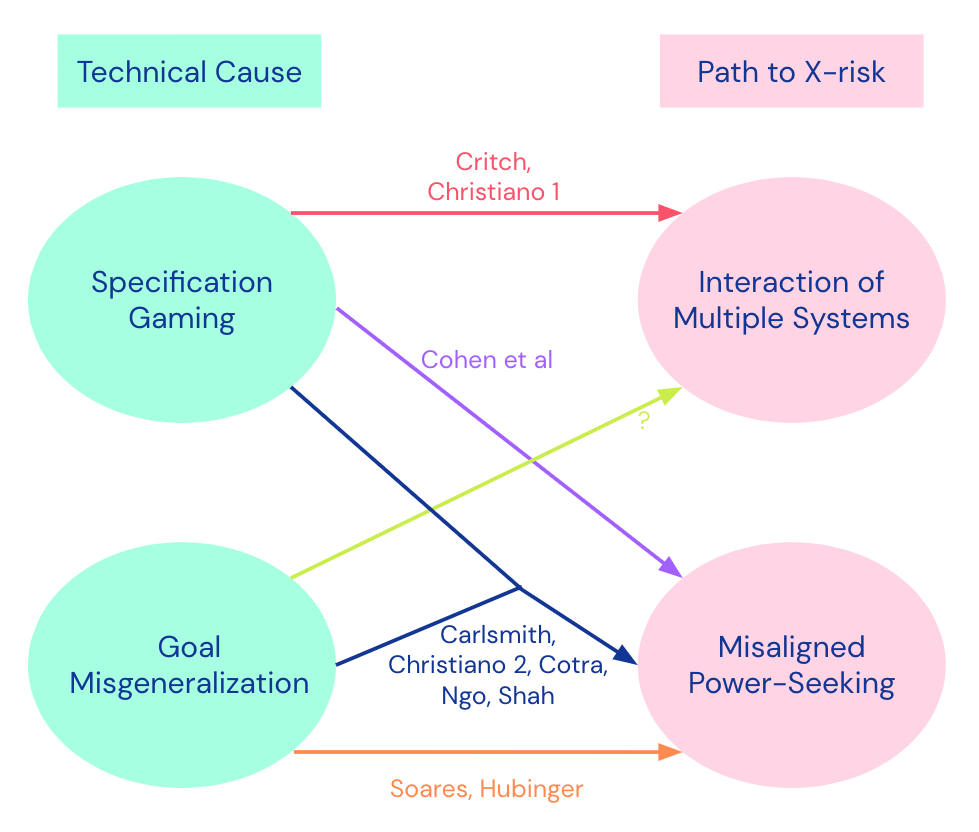
In terms of adding new threat models to the categorization, the main one that comes to mind is Deep Deceptiveness, which I would summarize as “non-deceptiveness is anti-natural / hard to disentangle from general capabilities”. I would probably put this under “SG → MAPS”, assuming an irreducible kind of specification gaming where it’s very difficult (or impossible) to distinguish deceptiveness from non-deceptiveness (including through feedback on the model’s reasoning process). Though it could also be GMG where the “non-deceptiveness” concept is incoherent and thus very difficult to generalize well.
Refining the Sharp Left Turn was an attempt to understand this threat model better (or at all) and make it a bit more concrete. I still endorse the breakdown of claims in this post.
The post could be improved by explicitly relating the claims to the “consensus” threat model summarized in Clarifying AI X-risk. Overall, SLT seems like a special case of this threat model, which makes a subset of the SLT claims:
- Claim 1 (capabilities generalize far) and Claim 3 (humans fail to intervene), but not Claims 1a/b (simultaneous / discontinuous generalization) or Claim 2 (alignment techniques stop working).
- It probably relies on some weaker version of Claim 2 (alignment techniques failing to apply to more powerful systems in some way) is needed for deceptive alignment to arise, e.g. if our interpretability techniques fail to detect deceptive reasoning. However, I expect that most ways this could happen would not be due to the alignment techniques being fundamentally inadequate for the capability transition to more powerful systems (the strong version of Claim 2 used in SLT).